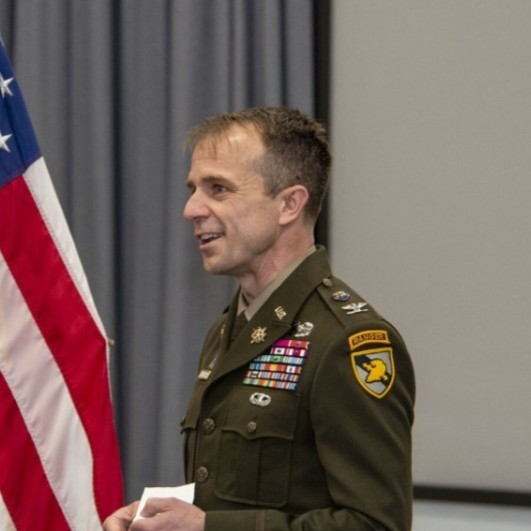
Colonel, US Army
Associate Professor, Department of Mathematical Sciences
United States Military Academy
Office: 229 Thayer Hall
Email: nicholas.clark@westpoint.edu
Curriculum Vita
Bio
Dr. Nicholas Clark is an Associate Professor at the University of St. Thomas in Saint Paul, Minneota. His areas of expertise include spatio-temporal statistical modeling, application of statistics and data science to problems in defense and national security, and program development and assessment of undergraduate data science programs.
Dr. Clark has published across a wide range of topics and is currently focusing on identifiability issues in spatio-temporal models for point process data. He also is currently a Co-PI for an NSF funded project entitled SCORE with Data and currently serves as an Editor for the online module subimssions to the project. This projects creates case-based learning opportunities involving sports data for undergraduate students in data science courses. The project involves academics and industry professionals in sports.
Prior to serving on faculty at UST, Nick was at the United States Military Academy at West Point for 6 years where he helped establish and led their Applied Statistics and Data Science Program.
While at West Point, Dr. Clark was instrumental in creating the US Army's Data Literacy 101 education program and has also done work on developing an effective assessment process for data literacy education across large organizations.
As an educator, Dr. Clark has over 8 years experience teaching at the undergraduate level. He has taught over 25 sections of 7 different undergraduate courses. He also is a trained ABET program evaluator for data science programs and is a leader in the development of undergraduate data science curricula.
Selected Recent Publications
- Assessment and Continuous Improvement of an Undergraduate Data Science Program Abstract In recent years, there has been an explosion in the growth of undergraduate statistics and data science programs across the US. Simultaneously, there has been clear guidance written on curriculum development for both data science (De Veaux et al.) and statistics (Carver et al.) programs. While this was occurring, ABET (now simply an acronym, but previously standing for the Accreditation Board for Engineering and Technology), in coordination with organizations such as the American Statistical Association, developed accreditation criteria for Data Science programs. In this article, we discuss our journey through ABET accreditation and discuss how adopting ABET processes for continuous improvement strengthens a program’s assessment process. We share best practices for working across multiple departments to collect data not only on individual courses, but also on the program as a whole. While the framework presented was initially established to support ABET accreditation, we argue that a properly executed program assessment should occur regardless of whether or not an institution is seeking ABET accreditation for their data science program. Throughout this article, we also discuss the extent to which ABET requirements naturally fit within our program’s existing goals, including an assessment of how ABET requirements align with major ideas in the field of data science education.
- Extended Laplace Approximation for Self-Exciting Spatio-Temporal Modles of Count Data Abstract Self-exciting models are statistical models of count data where the probability of an event occurring is influenced by the history of the process. In particular, self-exciting spatio-temporal models allow for spatial dependence as well as temporal self-excitation. For large spatial or temporal regions, however, the model leads to an intractable likelihood. An increasingly common method for dealing with large spatio-temporal models is by using Laplace approximations (LA). This method is convenient as it can easily be applied and is quickly implemented. However, as we will demonstrate in this manuscript, when applied to self-exciting Poisson spatial–temporal models, Laplace Approximations result in a significant bias in estimating some parameters. Due to this bias, we propose using up to sixth-order corrections to the LA for fitting these models. We will demonstrate how to do this in a Bayesian setting for self-exciting spatio-temporal models. We will further show there is a limited parameter space where the extended LA method still has bias. In these uncommon instances we will demonstrate how a more computationally intensive fully Bayesian approach using the Stan software program is possible in those rare instances. The performance of the extended LA method is illustrated with both simulation and real-world data
- Machine Learning Modeling Practices to Support the Principles of AI and Ethics in Nutrition Nutrition research is relying more on artificial intelligence and machine learning models to understand, diagnose, predict, and explain data. While artificial intelligence and machine learning models provide powerful modeling tools, failure to use careful and well-thought-out modeling processes can lead to misleading conclusions and concerns surrounding ethics and bias.